Artificial Intelligence for Medicine, Health, & Nutrition
For the Stoa A.I. topic (Resolved: The USFG should substantially reform the use of Artificial Intelligence technology), can improved A.I. advance health and medical care, including cancer and other disease research and therapies? And could A.I. help decode the dynamics of healthy microbiomes and how our microbes respond to dietary changes, stress, and exercise?
If A.I. reforms are called for, how could reforming government policies and programs be helpful (according to the affirmative), or unhelpful (according to the negative)? A.I. has improved diagnostic abilities, for example, in detecting early cancer: Artificial Intelligence Advances Breast Cancer Detection (Health IT Analytics, October 7, 2021). But improved early detection of breast cancers puts additional reliance on effective treatment of early breast cancer.
The tragic history of an overly-enthusiastic surgeon promoting radical mastectomies, radiologists advocating radiation, and pharmaceutical companies promoting chemotherapy. How successful have these interventions been since the “War on Cancer” was launched in the Nixon Administration? See, for example: War on Cancer Progress Depends on Market Innovation (Catalyst, March 23, 2019)
Could new A.I. tools offer paths to better outcomes? For discussion see: Might Cancer Be a Metabolic Disease? (Cancer Commons, October 31, 2018)
However, when new federal funding is provided for A.I. tools to diagnose or treat cancer, it will flow to supporters of established cancer therapies. Similarly, government funding flows to established theories about the what causes obesity (energy balance theory), diabetes, and cardiovascular disease. If these diseases and chronic conditions are metabolic in nature, and can be effectively treated with dietary changes, new government-funded A.I. tools will likely miss this and be programmed to favor institutionally-backed surgical, medical device, radiation, and pharmacological approaches.
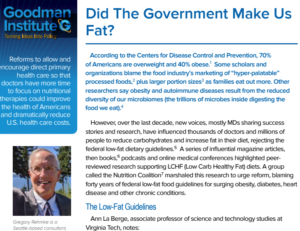
This also applies to the Stoa LD topic on restraint vs. scientific advances with biomedical devices. Federal research grants help fund development of biomedical engineering and new medical devices (also funded by medical device companies). Such research funding is scarce for testing nutritional interventions (low-carb and keto diets, for example), which in practice have been effective in improving metabolic health and putting type 2 diabetes into remission. For more see my Goodman Institute study, Did the Government Make Us Fat? (February 24, 2022)
Artificial or Fake Intelligence?
There are major problems and dangers with artificial intelligence, beginning with the phrase itself. A.I. skeptics note that technology language matters. The first motorcycles weren’t called “artificial horses” and the Wright Brothers didn’t invent an “artificial bird.” ‘The Age of AI and Our Human Future’ Review: Where Is My Thinking Machine?, Wall Street Journal, December 1, 2022 explains:
In 1950, a year before the world’s first commercial computer was delivered, the pioneering computer scientist Alan Turing published an essay asking “Can machines think?” A few years later another pioneer, John McCarthy, coined the term “artificial intelligence”—AI. That’s when the trouble began.
The sloppy but clever term “artificial intelligence” has fueled misdirection and misunderstanding: Imagine, in the 1920s, calling the car an artificial horse, or the airplane an artificial bird, or the electric motor an artificial waterwheel.
Artificial Intelligence has great potential for medical… disasters. Gary Smith, author of The AI Delusion, gives many examples of machine learning misapplied. In Why Was IBM Watson a Flop in Medicine? Robert J. Marks and Gary Smith discuss how IBM believed Watson:
…would be able to revolutionize medical care, health care. And it’s not worked because they could look up symptoms of various diseases and they could look up cures for various diseases and they could look up medical articles. But they don’t understand which are more meaningful than others, which medical articles are reasonable and which are bull and so a lot of doctors have become disillusioned with Watson. And a lot of hospitals have literally pulled the plug.
Of course IBM Watson is one of many commercial A.I. products. It should be no surprise that some are more capable and successful in the marketplace than others. At Economic Thinking debate workshops I’ve profiled Alphabet’s DeepMind, in part because former homeschool debater Andrew Trask is involved. Andrew is also Creator and Leader of OpenMined. He discusses his work and passion in this YouTube video Privacy Preserving AI (Andrew Trask) | MIT Deep Learning Series and a shorter video here: Privacy Preserving AI – Andrew Trask, OpenMined.
By using machine learning algorithms to search protected data sets, researchers can explore health data without violating privacy regulations, searching for connections between drugs, food additives, microbiomes, or pollutions correlated with particular cancers or other diseases.
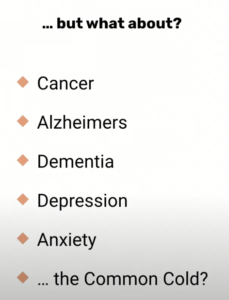
However, there are challenges with machine learning based on weak premises. For example, at right is a list of medical conditions currently off-limits to A.I. because the key information is locked up by state and federal medical privacy regulations. Privacy preserving A.I. could review this information without moving it out of regulatory protected databases (in hospitals and health care companies, for example). A.I. programs return the key information without compromising privacy.
Consider an A.I. machine learning program to check all who suffered severe and critical Covid and compare that to their vitamin D levels during and before their times in the hospital. See, for example, Dr. John Campbell’s Vitamin D, Strong evidence from Israel video (March 3, 2022), reviewing this recent paper: Pre-infection 25-hydroxyvitamin D3 levels and association with severity of COVID-19 illness (PLOS One, February 3, 2022).
Privacy preserving A.I. searching Covid and vitamin D levels might discover key nutrients, drugs, or other factors that increase or decrease risk.
Similar challenges exist with A.I. efforts to find causes or develop drugs or therapies for obesity, diabetes, and cardiovascular disease. Since obesity greatly increases the risk for cancer (and other diseases), and type 2 diabetes greatly increases the risk of Alzheimers (often called “type 3 diabetes”), finding new correlations should help.
But again, there are disagreements about even the nature of obesity and type 2 diabetes. Is type 2 diabetes a chronic and irreversible condition? It was thought to be so, and still is by many, and so is usually treated with various drugs to reduce blood glucose levels or in more severe cases, by daily injections of insulin. However, it turns out that simply reducing carbohydrates can improve blood glucose in days (or hours), and put type 2 diabetes into remission in a few weeks (for some 50% who try a low-carb or keto diet). So millions of funding for A.I. “cures” or new drugs for type 2 diabetes would be at the mercy (or command) of what algorithms are programmed to look for.
A.I. can also be deployed to further explore the human microbiome:
…a complex community of trillions of microorganisms (bacteria, archaea, viruses, as well as microbial eukaryotes such as fungi, protozoa and helminths), well-known to affect general health and homeostasis, e.g., by actively participating in human metabolism and regulating the immune system.
Applications of Machine Learning in Human Microbiome Studies… frontiers in Microbiology (19 February 2021)
I’ve written much more on nutritional science and disease, referencing studies, organizations, and institutional biases in federal health agencies and especially the federal dietary guideline. See Economic Thinking Nutrition/Public health posts, and posts on Normal Nutrition (a Substack site), with related podcasts at Normal Nutrition on Podbean and YouTube channel.